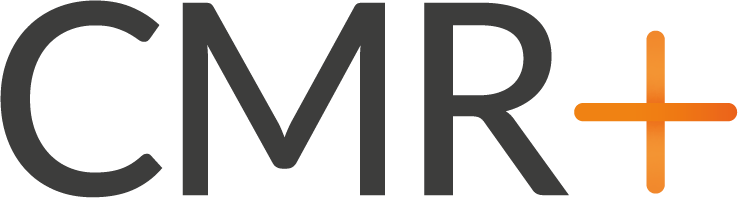
Auto QC for higher productivity
Really Intelligent Document Processing
Auto QC for higher productivity
CMR+ offers an Auto Quality Control (QC) feature that enhances productivity by automating the verification process and allowing users to customize confidence scores.
With Auto QC, CMR+ automatically assesses the accuracy and reliability of the processed documents based on predefined criteria. This eliminates the need for manual, time-consuming document reviews, resulting in higher productivity and faster turnaround times.
One key aspect of our Auto QC feature is the ability to customize confidence scores. Confidence scores are assigned to the extracted data, classifications, or predictions made by the ML models during document processing. Users can define confidence score thresholds that determine the level of confidence required for the system to automatically accept or flag the results.
By customizing confidence scores, users can establish quality control thresholds that align with their specific requirements and desired level of accuracy. For instance, if a user sets a high confidence score threshold, only the most accurate and reliable results will be automatically accepted, while results falling below the threshold will be flagged for manual review. This customization enables users to strike the right balance between automation and human oversight, optimizing productivity and maintaining control over the verification process.
Furthermore, CMR+ provides flexibility in defining quality control criteria beyond confidence scores. Users can establish additional validation rules or conditions based on specific data patterns, business rules, or regulatory requirements. These rules can be incorporated into the Auto QC process, ensuring that the processed documents meet the necessary criteria for accuracy, compliance, or other relevant factors.
The Auto QC feature is designed to be user-friendly and adaptable. Users can easily configure and fine-tune the quality control settings based on their evolving needs. Additionally, the platform provides real-time feedback and monitoring capabilities, allowing users to track the quality control results and make adjustments as necessary.
By leveraging the Auto QC feature in CMR+, users can achieve higher productivity by automating the verification process. The ability to customize confidence scores and define quality control criteria ensures that the document processing workflows align with their specific accuracy requirements and compliance standards. Ultimately, this leads to improved efficiency, reduced manual effort, and enhanced productivity in document processing tasks.
See It For Yourself
Don’t take our word for it. Nothing beats a demo tailored to your business needs.
The CMR
Process
Input Sources & Document Types
- Auto Ingestion
- Image
- Jpeg, TIFF, PDF
- MS Word
- RPA
- DMS
Document Optimisation & Indexing
- Noise Reduction
- Orientation/Skew Correction
- Background suppression
- Classification & Indexing
Data
Extraction
- Structured
- Un-structured
- Natural Language
- Handwritten
Data
Enrichment
- Business Rules
- Look ups
- API
Human in the Loop
- Verification
- Training
Machine Learning
Reports & Analytics
Workflow Management
Queue, exeption
& approval management
Data Transport & Mobilisation
RPA, APIs & Micro-services
Client Systems
Training as a part of the workflow
CMR+ integrates training as a part of the workflow, allowing the ML models to learn and improve as it processes more and more documents. By incorporating feedback, corrections, and iterative learning, the models continually refine their algorithms and enhance their accuracy. This capability ensures that CMR+ provides increasingly accurate and efficient document processing results over time, aligning closely with your organization’s specific needs and evolving document patterns.